Improved Brain Decoder Holds Promise for Communication in People With Aphasia
Restoring some language for aphasia sufferers, like Bruce Willis and a million other Americans, could involve AI.
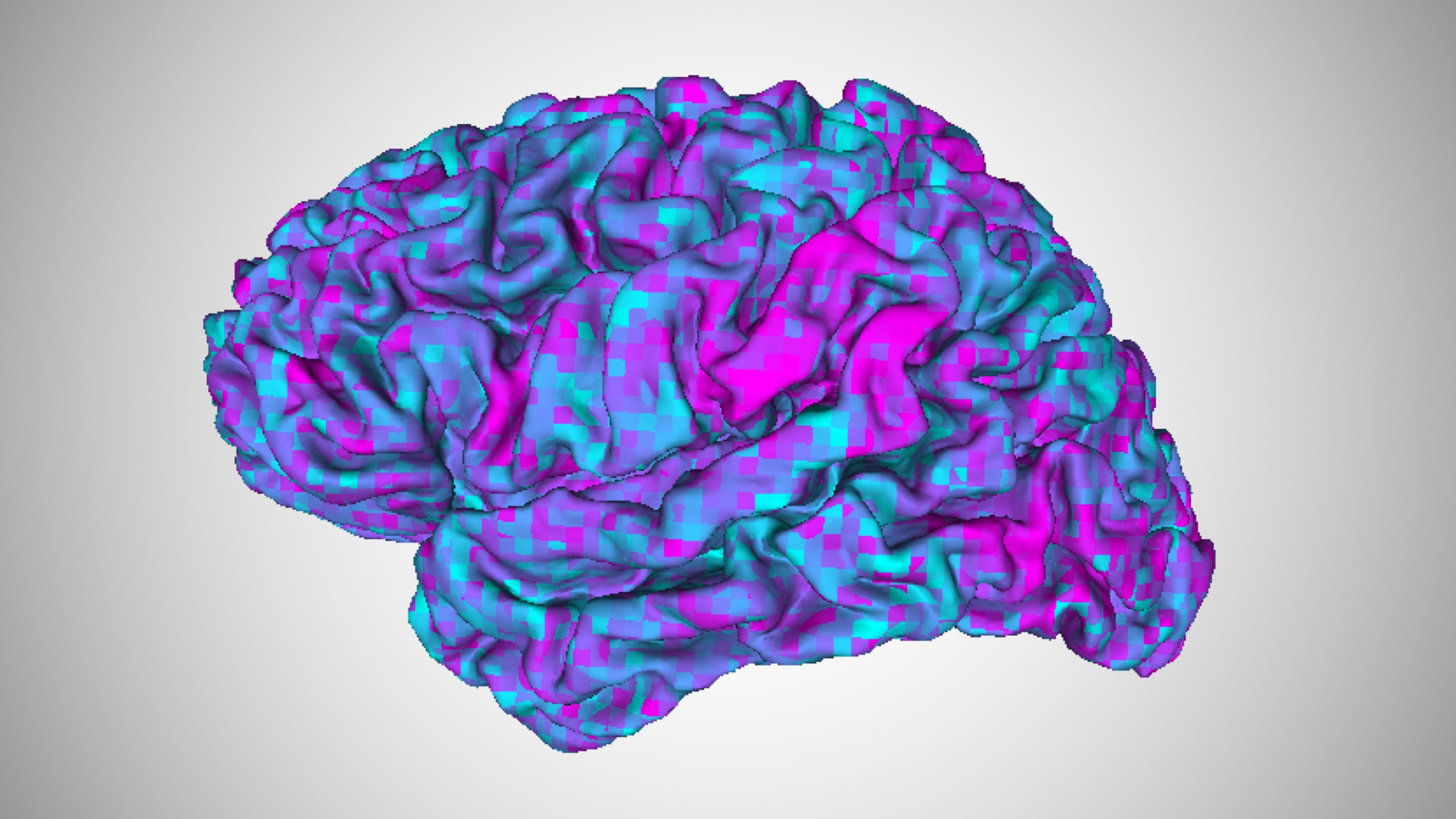
Brain activity like this, measured in an fMRI machine, can be used to train a brain decoder to decipher what a person is thinking about. In this latest study, UT Austin researchers have developed a method to adapt their brain decoder to new users far faster than the original training, even when the user has difficulty comprehending language. Credit: Jerry Tang/University of Texas at Austin.
People with aphasia — a brain disorder affecting about a million people in the U.S. — struggle to turn their thoughts into words and comprehend spoken language.
A pair of researchers at The University of Texas at Austin has demonstrated an AI-based tool that can translate a person’s thoughts into continuous text, without requiring the person to comprehend spoken words. And the process of training the tool on a person’s own unique patterns of brain activity takes only about an hour. This builds on the team’s earlier work creating a brain decoder that required many hours of training on a person’s brain activity as the person listened to audio stories. This latest advance suggests it may be possible, with further refinement, for brain computer interfaces to improve communication in people with aphasia.
“Being able to access semantic representations using both language and vision opens new doors for neurotechnology, especially for people who struggle to produce and comprehend language,” said Jerry Tang, a postdoctoral researcher at UT in the lab of Alex Huth and first author on a paper describing the work in Current Biology. “It gives us a way to create language-based brain computer interfaces without requiring any amount of language comprehension.”
In earlier work, the team trained a system, including a transformer model like the kind used by ChatGPT, to translate a person’s brain activity into continuous text. The resulting semantic decoder can produce text whether a person is listening to an audio story, thinking about telling a story, or watching a silent video that tells a story. But there are limitations. To train this brain decoder, participants had to lie motionless in an fMRI scanner for about 16 hours while listening to podcasts, an impractical process for most people and potentially impossible for someone with deficits in comprehending spoken language. And the original brain decoder works only on people for whom it was trained.
With this latest work, the team has developed a method to adapt the existing brain decoder, trained the hard way, to a new person with only an hour of training in an fMRI scanner while watching short, silent videos, such as Pixar shorts. The researchers developed a converter algorithm that learns how to map the brain activity of a new person onto the brain of someone whose activity was previously used to train the brain decoder, leading to similar decoding at a fraction of the time with the new person.
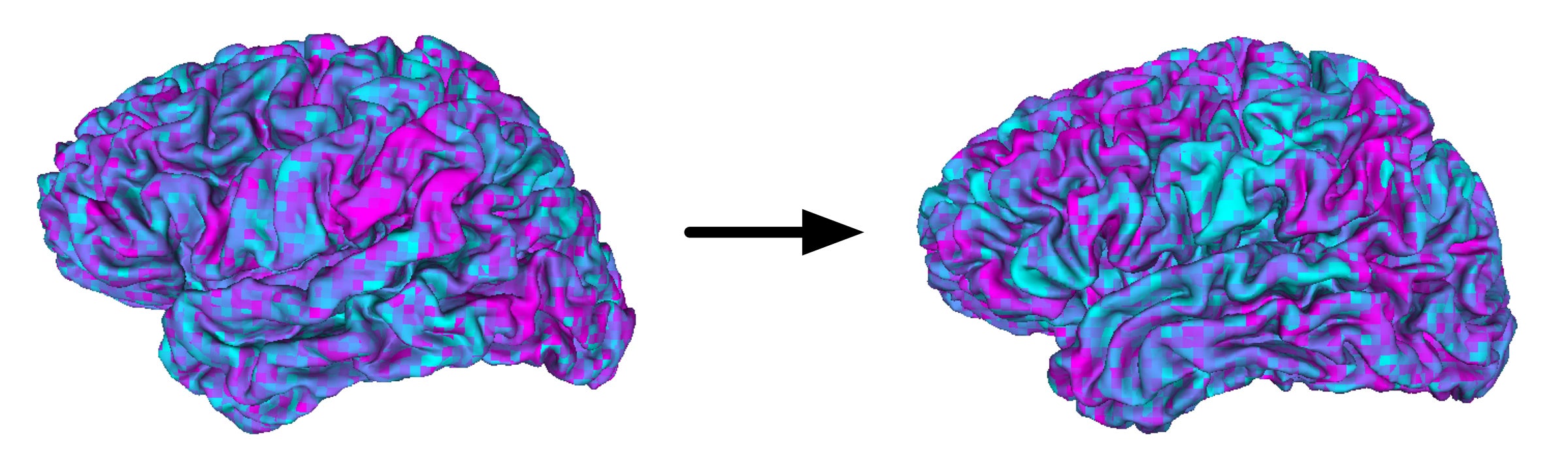
Brain activity from two people watching the same silent film. The UT Austin team developed a converter algorithm that transforms one person’s brain activity (left) into the predicted brain activity of the other person (right), which is a crucial step in adapting their brain decoder to a new subject. Credit: Jerry Tang/University of Texas at Austin.
Huth said this work reveals something profound about how our brains work: Our thoughts transcend language.
“This points to a deep overlap between what things happen in the brain when you listen to somebody tell you a story, and what things happen in the brain when you watch a video that’s telling a story,” said Huth, associate professor of computer science and neuroscience and senior author. “Our brain treats both kinds of story as the same. It also tells us that what we’re decoding isn’t actually language. It’s representations of something above the level of language that aren’t tied to the modality of the input.”
The researchers noted that, just as with their original brain decoder, their improved system works only with cooperative participants who participate willingly in training. If participants on whom the decoder has been trained later put up resistance — for example, by thinking other thoughts — results are unusable. This reduces the potential for misuse.
While their latest test subjects were neurologically healthy, the researchers also ran analyses to mimic the patterns of brain lesions in participants with aphasia and showed that the decoder was still able to translate into continuous text the story they were perceiving. This suggests that the approach could eventually work for people with aphasia.
They are now working with Maya Henry, an associate professor in UT’s Dell Medical School and Moody College of Communication who studies aphasia, to test whether their improved brain decoder works for people with aphasia.
“It’s been fun and rewarding to think about how to create the most useful interface and make the model-training procedure as easy as possible for the participants,” Tang said. “I’m really excited to continue exploring how our decoder can be used to help people.”
This work was supported by the National Institute on Deafness and Other Communication Disorders of the National Institutes of Health, the Whitehall Foundation, the Alfred P. Sloan Foundation and the Burroughs Wellcome Fund.
For more images and video b-roll visit: https://utexas.box.com/s/j1s3015cuv2wcl6jnweqm2t3s6ohvluv
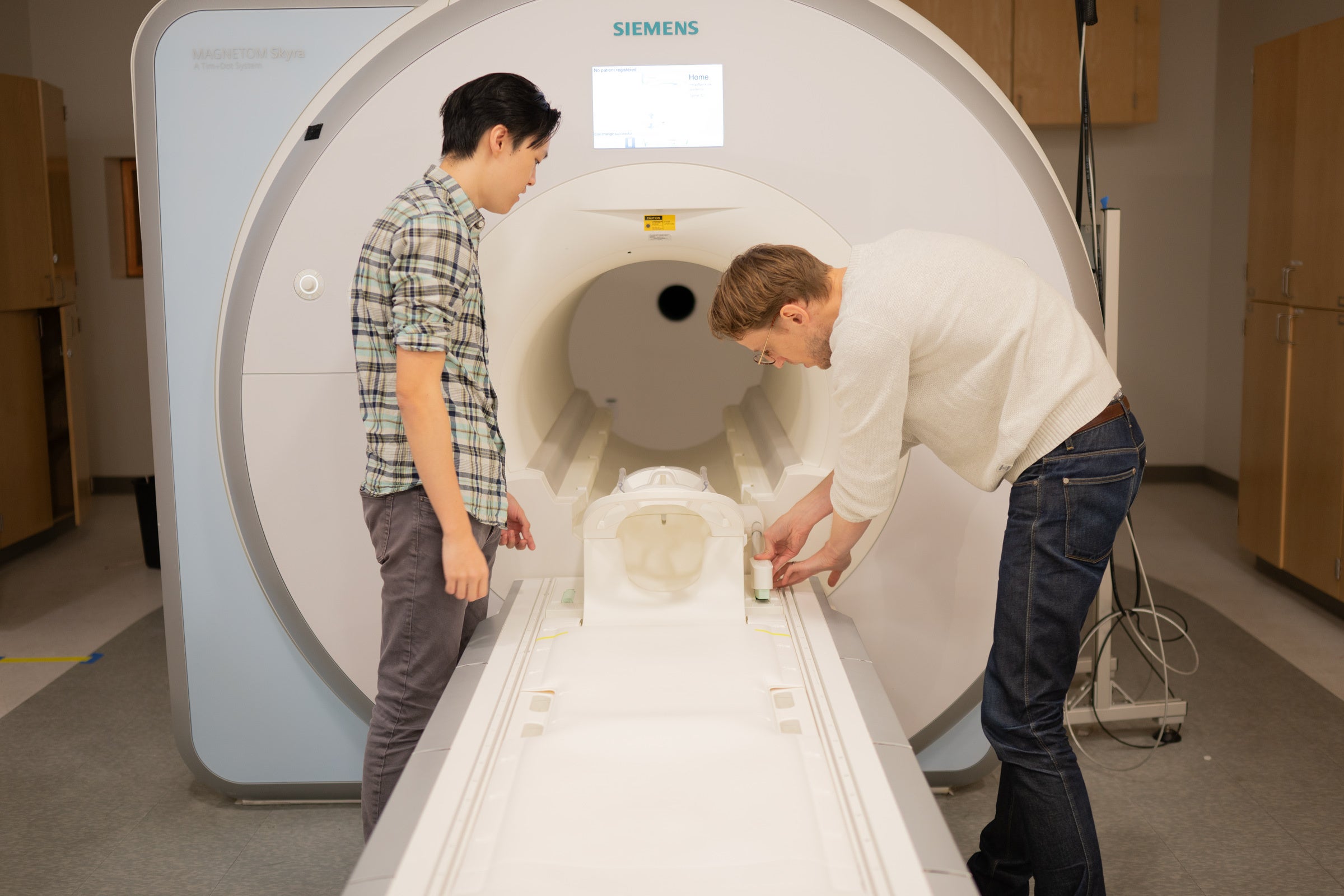
Jerry Tang (left) and Alex Huth (right) have demonstrated an AI-based tool that can translate a person’s thoughts into continuous text, without requiring the person to comprehend spoken words. Here they prepare the fMRI scanner to record a subject’s brain activity. Credit: Nolan Zunk/University of Texas at Austin.